Motivation
The importance of machine learning (ML) is increasing rapidly, especially in the recent past, while at the same time the technologies involved are becoming more mature. ML is used in many applications such as computer linguistics (CL), speech and object recognition, but also for vulnerability and malware detection.
An important reason that makes ML indispensable is that it allows us to make predictions and automate complex tasks without human intervention. Therefore, it is expected that machine learning will play an even more important role in almost all software applications in the near future, and will increasingly be integrated directly into various applications and devices to automate complex tasks.
The traditional approach to machine learning is built around a centralized infrastructure that is typically owned by one party. This infrastructure is used to store data and train predictive models. However, due to recent trends such as IoT, smart cities or autonomous driving, a typical ML setup has become much more complex. Not only the number of devices, applications and services that collect data to train predictive models has increased significantly but also the number of involved parties. To better support these setups, federated machine learning has become more important which allows to train predictive models in a decentralized manner.
The main idea of federated learning is that it enables devices to learn predictive models in a collaborative way while keeping all training data local. Federated machine learning is particularly useful in situations where the model is based on data collected and processed by a very large number of devices owned by different parties. Federated learning is thus used to minimize the costs and risks associated with processing sensitive data since training of models can directly happen on the end devices without moving sensitive data through the network.
In the typical federated learning setup today, a central server is still being used; i.e., the parties involved in the training process need to send their local model updates to a central server, which combines these updates into a global model. This approach to federated ML is called 'centralized federated machine learning'. However, this approach to federated ML has shown to open up many possible attacks to manipulate the predictive models (e.g., poisoning attacks) or even breach the privacy of participants.
Hence, solutions for efficient, secure and fully distributed federated learning architectures are essential, especially as learning algorithms are now used in many IT applications and autonomous systems in which attacks as outlined above could cause severe damages.
Researchers
Name | Contact | |
---|---|---|
![]() | Dr. rer. nat. Muhammad El-Hindi | muhammad.el-hindi@cs.tu-... S2|02 A309 |
Publications
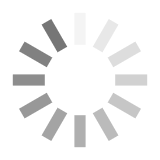
Error on loading data
An error has occured when loading publications data from TUbiblio. Please try again later.
-
{{ year }}
-
; {{ creator.name.family }}, {{ creator.name.given }}{{ publication.title }}.
; {{ editor.name.family }}, {{ editor.name.given }} (eds.); ; {{ creator }} (Corporate Creator) ({{ publication.date.toString().substring(0,4) }}):
In: {{ publication.series }}, {{ publication.volume }}, In: {{ publication.book_title }}, In: {{ publication.publication }}, {{ publication.journal_volume}} ({{ publication.number }}), ppp. {{ publication.pagerange }}, {{ publication.place_of_pub }}, {{ publication.publisher }}, {{ publication.institution }}, {{ publication.event_title }}, {{ publication.event_location }}, {{ publication.event_dates }}, ISSN {{ publication.issn }}, e-ISSN {{ publication.eissn }}, ISBN {{ publication.isbn }}, DOI: {{ publication.doi.toString().replace('http://','').replace('https://','').replace('dx.doi.org/','').replace('doi.org/','').replace('doi.org','').replace("DOI: ", "").replace("doi:", "") }}, Official URL, {{ labels[publication.type]?labels[publication.type]:publication.type }}, {{ labels[publication.pub_sequence] }}, {{ labels[publication.doc_status] }} - […]
-
Number of items in this list: >{{ publicationsList.length }}
Only the {{publicationsList.length}} latest publications are displayed here.