Paper accepted at PETS 2022
2021/12/07
In this paper, TK and AI&ML researchers propose Label Leakage from Gradients (LLG), a novel attack to extract the labels of the users’ training data from their shared gradients in federated learning. The attack exploits the direction and magnitude of gradients to determine the presence or absence of any label. They mathematically and empirically demonstrate the validity of the attack under different settings. Moreover, empirical results show that LLG successfully extracts labels with high accuracy at the early stages of model training. They also discuss different defense mechanisms against such leakage.
Citation info:
- Aidmar Wainakh, Fabrizio Ventola, Till Müßig, Jens Keim, Carlos Garcia Cordero, Ephraim Zimmer, Tim Grube, Kristian Kersting, and Max Mühlhäuser. User-Level Label Leakage from Gradients in Federated Learning. In the annual Privacy Enhancing Technologies Symposium (PETS) 2022 [to appear].
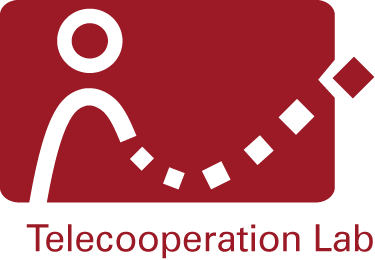