Neuro-Symbolic Modeling for City Scale Traffic Simulation
Bachelor Thesis, Master Thesis
In a large-scale urban environment, different navigation patterns can be extracted from data. These patterns are governed by numerous factors, such as social rules and the spatiotemporal context. Neuro-symbolic modeling integrates symbolic reasoning and machine-learning-based representations altogether. It represents, consequently, a promising approach to model large-scale motion patterns that are governed by rules and uncertainty at the same time.
Goal
Design and implement a neuro-symbolic program search module for multi-scale traffic simulation in virtual urban environments.
Requirements
- Prior knowledge in machine learning is required
- Hands-on programing experience with gaming engines is desirable (Unity, Unreal Engine, …)
- Eagerness to learn and work hard
Keywords
Traffic Simulation, Neuro-Symbolic Modeling, Deep Learning, Urban Environment
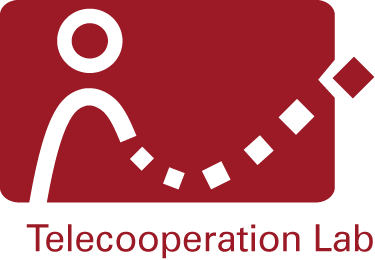