Motivation
While AI and here most often machine learning (ML) becomes more common as a tool for various security applications where data must be analysed, clustered or attributed, the security of the applied algorithms is often limited. Various research results in the past years show shortcomings of trained neural nets (NN) like robustness against targeted attacks. The risk of privacy loss is also discussed in the context of machine learning – potential leakage from trained NNs as well as de-anonymization in complex data sets with the help of machine learning. This leads to a lack of trust and acceptance by the public: ML is perceived as a risk and a threat, a mechanism to screen large parts of everyday life beyond control. Under these circumstances, utilizing the potential of ML for security solutions and other applications becomes challenging.
In IT security the usage of ML is already established in multiple domains today. SPAM detection is a well-known example where support vector machines try to distinguish wanted from unwanted emails. Author attribution combines natural language forensics and machine learning. Deep learning helps identifying illicit images and has improved malware detection as well as network intrusion detection.
Today ML algorithms and trained nets become the target of attacks. Various approaches try to mislead or influence ML-based decisions, requiring countermeasures of IT security protecting the core assets of ML. ML also becomes a tool of attackers. IT security needs to be prepared for attacks able to adapt more quickly to complex security measures, just like intrusion detection systems today aim to identify complex attacks with the help of ML. Adversarial machine learning will become more common in IT security. Whenever a security challenge can be described on the one hand with a relatively simple concept and on the other hand can be addressed by machine learning, the other side, be it defender or attacker, will use adversarial machine learning to efficiently identify weaknesses in the strategy of the other partner and deploy specialized attacks or defenses against it.
Goals
SenPAI addresses the subject of security and ML from two perspectives: The first perspective is improving the security of ML algorithms and systems based on ML. This does not include standard system security, which is a generic requirement for all IT systems. The focus is on security challenges that are especially and in some cases exclusively relevant to ML. The term “security” has to be seen in a broad sense here, as issues like privacy leakage or transparency of decisions shall also be addressed. The second perspective is application-centric. As CRISP has a focus on applied security solutions, in the project new security applications based on ML are to be developed and evaluated. These applications can and shall also utilize the security mechanisms developed in the technology-centric research projects and give a feedback on their usability and performance. The application-centric projects also can raise new challenges for the technology-centric projects. These projects will also potentially be Big Data projects due to their handling of complex data and their aim to efficiently derive information relevant for security matters from it.
The technology-centric projects will focus on research publications and PhD theses. Tools will be developed and implemented as well. The application-centric projects will focus on building demonstrators and discussing them with the public, governmental organizations and the industry.
Funding
This research work is funded from 2020 – 2023 by the German Federal Ministry of Education and Research and the Hessen State Ministry for Higher Education, Research and the Arts within their joint support of the National Research Center for Applied Cybersecurity ATHENE.
Partners
Fraunhofer SIT / MSF
Publications
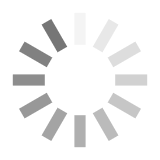
Error on loading data
An error has occured when loading publications data from TUbiblio. Please try again later.
-
{{ year }}
-
; {{ creator.name.family }}, {{ creator.name.given }}{{ publication.title }}.
; {{ editor.name.family }}, {{ editor.name.given }} (eds.); ; {{ creator }} (Corporate Creator) ({{ publication.date.toString().substring(0,4) }}):
In: {{ publication.series }}, {{ publication.volume }}, In: {{ publication.book_title }}, In: {{ publication.publication }}, {{ publication.journal_volume}} ({{ publication.number }}), ppp. {{ publication.pagerange }}, {{ publication.place_of_pub }}, {{ publication.publisher }}, {{ publication.institution }}, {{ publication.event_title }}, {{ publication.event_location }}, {{ publication.event_dates }}, ISSN {{ publication.issn }}, e-ISSN {{ publication.eissn }}, ISBN {{ publication.isbn }}, DOI: {{ publication.doi.toString().replace('http://','').replace('https://','').replace('dx.doi.org/','').replace('doi.org/','').replace('doi.org','').replace("DOI: ", "").replace("doi:", "") }}, Official URL, {{ labels[publication.type]?labels[publication.type]:publication.type }}, {{ labels[publication.pub_sequence] }}, {{ labels[publication.doc_status] }} - […]
-
Number of items in this list: >{{ publicationsList.length }}
Only the {{publicationsList.length}} latest publications are displayed here.