Motivation
Dictionaries are an essential resource in many domains of research, education, and natural language processing (NLP). Nowadays, dictionaries are digital resources, often accessible through websites and webservices, thus being a part of the portfolio of modern e-Research technologies and infrastructures.
One crucial part of dictionaries are example sentences which illustrate use cases of for a specific lemma. Research on dictionary use has shown that users even tend to refer to (good) examples instead of consulting the rather complex descriptions of word grammar in dictionaries. However, dictionaries of contemporary languages have to meet two important requirements: being comprehensive and up-to-date; which means that newly emerging words need to be listed including their senses and usages. To do so, lexicographers are required to select usage examples from a large list of candidate sentences.
Recent studies show that existing systems for automatic evaluation of dictionary examples are good in identifying bad ones, but not able to provide a fine-grained scale for potentially good dictionary examples. In this project, we develop a novel system which eases the work of lexicographers by interactively assessing the goodness and diversity of dictionary examples.
Goals
The key features of our dictionary example selection system include:
- Providing good estimates for the goodness of an example sentence
- Considering the diversity of yet-to-be-selected examples wrt. to the already selected set of examples
- Interactive adaptation of our system based on a lexicographer's feedback
We unite all features into an interactive lexicographer interface which is adaptable to any language and use case (e.g., the creation of second language learning dictionaries).
Method
The progress of the project will be led by an iterative methodology that encompasses the following:
- Corpus – together with lexicographers from the DWDS we compile a corpus consisting of pairwise annotations between dictionary example sentences and increase it successively.
- Interactive preference learning – Utilizing recent insights from preference learning and sentence classification with contextualized language models, we develop approaches which interactively learn from a lexicographer's feedback to automatically suggest better and more diverse dictionary examples.
- Crowd-sourced feedback – We additionally incorporate feedback from lay-users of the dictionary as an additional signal for our trained models to further improve the quality of automatically extracted dictionary examples.
Team
- Prof. Dr. Iryna Gurevych, Principal Investigator
Partners
This project is established in cooperation with the berlin-brandenburgische Akademie der Wissenschaften located in Berlin:
Funding
This project is funded by (German Research Foundation). Deutsche Forschungsgemeinschaft
Publications
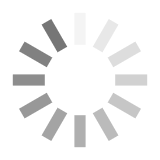
Error on loading data
An error has occured when loading publications data from TUbiblio. Please try again later.
-
{{ year }}
-
; {{ creator.name.family }}, {{ creator.name.given }}{{ publication.title }}.
; {{ editor.name.family }}, {{ editor.name.given }} (eds.); ; {{ creator }} (Corporate Creator) ({{ publication.date.toString().substring(0,4) }}):
In: {{ publication.series }}, {{ publication.volume }}, In: {{ publication.book_title }}, In: {{ publication.publication }}, {{ publication.journal_volume}} ({{ publication.number }}), ppp. {{ publication.pagerange }}, {{ publication.place_of_pub }}, {{ publication.publisher }}, {{ publication.institution }}, {{ publication.event_title }}, {{ publication.event_location }}, {{ publication.event_dates }}, ISSN {{ publication.issn }}, e-ISSN {{ publication.eissn }}, ISBN {{ publication.isbn }}, DOI: {{ publication.doi.toString().replace('http://','').replace('https://','').replace('dx.doi.org/','').replace('doi.org/','').replace('doi.org','').replace("DOI: ", "").replace("doi:", "") }}, Official URL, {{ labels[publication.type]?labels[publication.type]:publication.type }}, {{ labels[publication.pub_sequence] }}, {{ labels[publication.doc_status] }} - […]
-
Number of items in this list: >{{ publicationsList.length }}
Only the {{publicationsList.length}} latest publications are displayed here.