Motivation
Open debates include so many arguments that sound decision making exceeds cognitive capabilities of the interested public or responsible experts. Until now, argument mining approaches typically map from a closed set of given texts into a formal argumentation model. However, this does not fully cater to the nature of open, ongoing debates because of the following challenges:
- (C1) Following a Continuous Debate: Participants in open, mass debates continuously introduce new arguments with novel aspects relevant to the debate topic. This leads to brittleness of state-of-the-art argument extractors, which are trained once and for all time.
- (C2) Dealing with Incomplete Arguments: Textual arguments are often incomplete because the participants in the debate can understand them based on common background or shared knowledge. Hence argument structures which are identified by current argument mining methods are often incomplete.
- (C3) Establishing Open Knowledge for Argumentation: Interpreting and understanding textual arguments requires additional facts and common knowledge which are often absent in existing knowledge graphs.
Goals
This project aims at investigating computational methods that i) continuously improve their capability to recognize arguments in ongoing debates, ii) align incomplete arguments with previous arguments and enrich them with automatically acquired background knowledge, and iii) constantly extend semantic knowledge bases with information required to understand arguments. Our goal is to arrive at an Open Argument Mining approach that is open with respect to new arguments emerging in ongoing debates, open to consider and contextualize incomplete arguments, and open to continuously acquire and extend knowledge required to understand such arguments.
Partners
This project was established in cooperation with in Koblenz. WeST: Institute for Web Science and Technologies
Publications
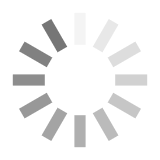
Error on loading data
An error has occured when loading publications data from TUbiblio. Please try again later.
-
{{ year }}
-
; {{ creator.name.family }}, {{ creator.name.given }}{{ publication.title }}.
; {{ editor.name.family }}, {{ editor.name.given }} (eds.); ; {{ creator }} (Corporate Creator) ({{ publication.date.toString().substring(0,4) }}):
In: {{ publication.series }}, {{ publication.volume }}, In: {{ publication.book_title }}, In: {{ publication.publication }}, {{ publication.journal_volume}} ({{ publication.number }}), ppp. {{ publication.pagerange }}, {{ publication.place_of_pub }}, {{ publication.publisher }}, {{ publication.institution }}, {{ publication.event_title }}, {{ publication.event_location }}, {{ publication.event_dates }}, ISSN {{ publication.issn }}, e-ISSN {{ publication.eissn }}, ISBN {{ publication.isbn }}, DOI: {{ publication.doi.toString().replace('http://','').replace('https://','').replace('dx.doi.org/','').replace('doi.org/','').replace('doi.org','').replace("DOI: ", "").replace("doi:", "") }}, Official URL, {{ labels[publication.type]?labels[publication.type]:publication.type }}, {{ labels[publication.pub_sequence] }}, {{ labels[publication.doc_status] }} - […]
-
Number of items in this list: >{{ publicationsList.length }}
Only the {{publicationsList.length}} latest publications are displayed here.