Motivation
Researchers in NLP have devoted significant resources to the creation of more powerful machine learning models for question answering (QA), and the collection of high-quality QA datasets. Combined with the recent breakthroughs by large pretrained language models, we have witnessed a rapid progress in the field, also across many different kinds of QA. This has led to situations where state-of-the-art models become obsolete only after a few months when they have been developed.
Therefore, it is essential for researchers to explore, compare, and combine these models as quickly as possible to identify the strengths and weaknesses of the current state of the art. When QA systems are accompanied by tools to analyse intermediate results of models and pipelines, they can also provide important insights to understand the ‘black box’ of modern neural networks.
Even though there exists a large variety of QA systems and frameworks, present approaches cannot be easily extended with additional kinds of QA that use different types of data sources, models, pipelines, and answer types. For instance, there exists no framework that allows researchers to easily integrate a skill to perform cQA and a skill to perform yes/no QA for claim validation within the same platform, which can then easily inter-operate—e.g., to validate claims that are being made in the user-generated answers, found in cQA. This considerably limits their applicability and re-use across the diverse, rapidly progressing area of QA research, making it infeasible for researchers to quickly integrate novel models and QA pipelines.
Goals
In this project, we aim to create a flexible, scalable and interpretable QA platform to enable researchers to:
- Share their custom QA agents by integrating them to our platform using easy-to-use common interfaces,
- Study the strengths and weaknesses of existing models by comparing them on a wide range of tasks and datasets that are already provided within our framework,
- Explore the existing models and datasets to answer more specific research questions using integrated interpretability tools.
For public users, we also aim to develop a QA demonstrator that can combine answers of different QA agents to provide better answers.
Team
- Prof. Dr. Iryna Gurevych, Principal Investigator
- Haritz Puerto, MSc, Doctoral Researcher
- Tim Baumgärtner, MSc, Doctoral Researcher
- Rachneet Sadeva, MSc, Doctoral Researcher
Funding
This project is funded by the Deutsche Forschungsgemeinschaft (German Research Foundation).
Publications
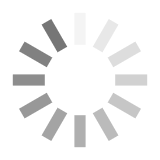
Error on loading data
An error has occured when loading publications data from TUbiblio. Please try again later.
-
{{ year }}
-
; {{ creator.name.family }}, {{ creator.name.given }}{{ publication.title }}.
; {{ editor.name.family }}, {{ editor.name.given }} (eds.); ; {{ creator }} (Corporate Creator) ({{ publication.date.toString().substring(0,4) }}):
In: {{ publication.series }}, {{ publication.volume }}, In: {{ publication.book_title }}, In: {{ publication.publication }}, {{ publication.journal_volume}} ({{ publication.number }}), ppp. {{ publication.pagerange }}, {{ publication.place_of_pub }}, {{ publication.publisher }}, {{ publication.institution }}, {{ publication.event_title }}, {{ publication.event_location }}, {{ publication.event_dates }}, ISSN {{ publication.issn }}, e-ISSN {{ publication.eissn }}, ISBN {{ publication.isbn }}, DOI: {{ publication.doi.toString().replace('http://','').replace('https://','').replace('dx.doi.org/','').replace('doi.org/','').replace('doi.org','').replace("DOI: ", "").replace("doi:", "") }}, Official URL, {{ labels[publication.type]?labels[publication.type]:publication.type }}, {{ labels[publication.pub_sequence] }}, {{ labels[publication.doc_status] }} - […]
-
Number of items in this list: >{{ publicationsList.length }}
Only the {{publicationsList.length}} latest publications are displayed here.