Motivation
The (responsible) use of artificial intelligence (AI) is currently being discussed in many industry sectors. Especially production-driven solutions, managed via a shop floor management system require a high amount of effort to properly document processes during manufacturing and may substantially profit from AI-driven solutions.
However, a lot of information w.r.t. problems and appropriate solutions during production remains hidden as they are often not communicated via official channels but more convenient, unstructured channels. To grant companies access to such hidden information, this project investigates the use of AI-driven methods to automatically extract and utilize relevant information from unstructured texts like chatlogs.
This requires a close liaison between production- and text analysis and thus, interdisciplinary collaboration. An optimal premise to tackle the responsible transfer of knowledge from automated text analytic algorithms to the explicit use case of improving the shop floor management is provided by the collaboration of researchers from the institute of Produktionsmanagement, Technologie und Werkzeugmaschinen (PTW) lead by Prof. Dr. Metternich and the Ubiquitous Knowledge Processing (UKP) Lab lead by Prof. Dr. Gurevych.
Methods and Workflow
Figure 1 shows the overall workflow of this project. For workers in a factory environment it is often more convenient to simply message a colleague if an error occurs. However, as error protocols are only filled out after a shift, less severe errors as shown in figure 1 may get lost. This may lead to severe errors later on, for example, if a frequent error indicating the necessity for machine maintenance remains unnoticed by the system. Automatically extracting relevant entities and pre-filling error protocols serves two purposes. First, to remind the worker that an error occurred during work and needs to be documented. Second, to alleviate their work by asking them to only provide corrections to the error protocol.
For recognizing entities and their relations, we investigate state-of-the-art named entity recognition models and their transfer to low-resource scenarios. Co-reference resolution models furthermore assist in identifying important relations between different entities. We furthermore investigate the impact of employing AI-driven methods in the factory environment and identify further use cases of such methods. One further goal of this project is to serve as a guiding project towards the responsible use of AI-driven solutions within industrial production facilities. As such, one important factor is the collection of data produced by employees during their working hours.
Data Collection
To collect appropriate data for training our neural network models, we employ an open source messaging application in a simulated factory environment. To protect the privacy of the participants it is essential that data is collected anonymously. We thus implement guidelines in consultation with the ethics committee of TU Darmstadt to ensure that data cannot be traced back to individual participants.
Goals
- Identifying requirements for the responsible use of chatlog analysis methods for solving problems in the factory environment
- Automated extraction and modeling of relevant entities from unstructured data for use in a factory environment
- Creating workflows for efficiently implementing such approaches in industry
Team
UKP
- Prof. Dr. Iryna Gurevych
- Ji-Ung Lee
- Prof. Dr. Joachim Metternich
- Marvin Müller
- Nicholas Frick
Funding
This project was funded by the Europäischen Fonds für Regionale Entwicklung (EFRE) [Eng. Translation: European Fonds for Regional Development] and the Hessische Staatskanzlei – Ministerin für Digitale Strategie und Entwicklung [Eng. Translation: Hessian Ministry for Digital Strategy and Development].
Publications
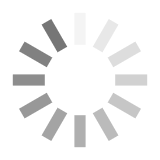
Error on loading data
An error has occured when loading publications data from TUbiblio. Please try again later.
-
{{ year }}
-
; {{ creator.name.family }}, {{ creator.name.given }}{{ publication.title }}.
; {{ editor.name.family }}, {{ editor.name.given }} (eds.); ; {{ creator }} (Corporate Creator) ({{ publication.date.toString().substring(0,4) }}):
In: {{ publication.series }}, {{ publication.volume }}, In: {{ publication.book_title }}, In: {{ publication.publication }}, {{ publication.journal_volume}} ({{ publication.number }}), ppp. {{ publication.pagerange }}, {{ publication.place_of_pub }}, {{ publication.publisher }}, {{ publication.institution }}, {{ publication.event_title }}, {{ publication.event_location }}, {{ publication.event_dates }}, ISSN {{ publication.issn }}, e-ISSN {{ publication.eissn }}, ISBN {{ publication.isbn }}, DOI: {{ publication.doi.toString().replace('http://','').replace('https://','').replace('dx.doi.org/','').replace('doi.org/','').replace('doi.org','').replace("DOI: ", "").replace("doi:", "") }}, Official URL, {{ labels[publication.type]?labels[publication.type]:publication.type }}, {{ labels[publication.pub_sequence] }}, {{ labels[publication.doc_status] }} - […]
-
Number of items in this list: >{{ publicationsList.length }}
Only the {{publicationsList.length}} latest publications are displayed here.