UKP/AIPHES paper wins Best Paper Award at EMNLP 2017
2017/08/15
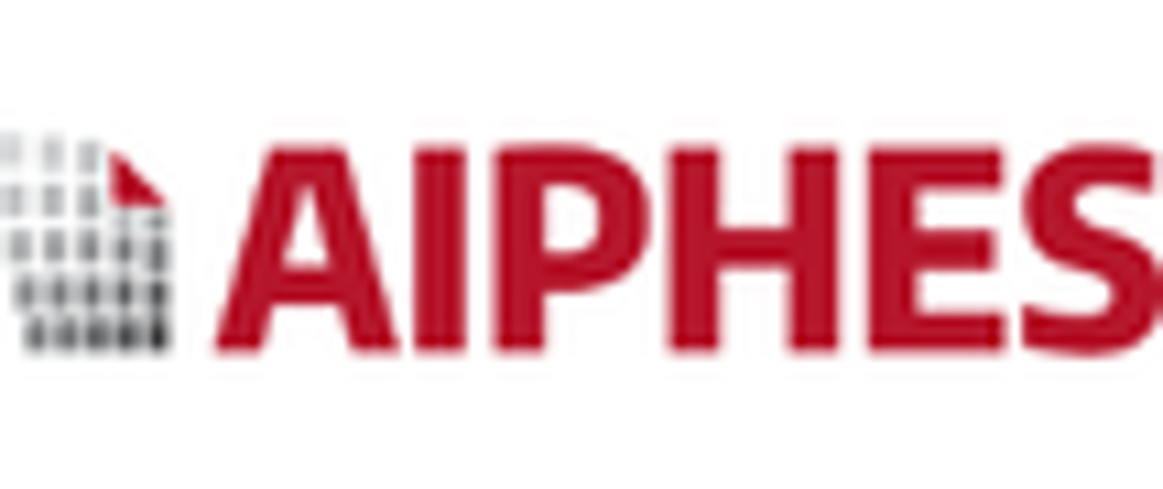
Bringing Structure into Summaries: Crowdsourcing a Benchmark Corpus of Concept Maps by Tobias Falke and Iryna Gurevych has been awarded Best Resource/Application Paper at the Conference on Empirical Methods in Natural Language Processing 2017 in Copenhagen.
The main contribution of the paper is a new, large-scale benchmark corpus of structured summaries of document collections in the form of concept maps. Concept maps – graphs depicting key concepts and relationships between them – can be used to concisely represent important information and bring structure into large document collections. But although multiple studies have confirmed the usefulness of this type of representation in the past, research on methods that automatically extract concept maps from texts is rare. The paper and the corpus are therefore a crucial step to increase attention for this task and enable future work.
The following example shows a part of a concept map on the topic of student loans:
The new corpus provides manually created reference concept maps that summarize heterogeneous collections of web documents on educational topics. Together with several proposed evaluation metrics, it can be used to develop and compare different methods that create concept map summaries.
Further, the paper presents a new methodology that has been used to create the corpus. Using a multi-step process that combines automatic preprocessing, scalable crowdsourcing and manual expert annotations, the complex annotation task could be performed efficiently despite the large document clusters that have been summarized. As a crucial step, the process contains a novel crowdsourcing approach that makes it possible to determine important elements in large document collections with the help of hundreds of crowd-workers.
For further details, please refer to the following resources:
One document cluster of the corpus can also be explored using the following web demo, which is part of : another UKP/AIPHES publication at EMNLP 2017
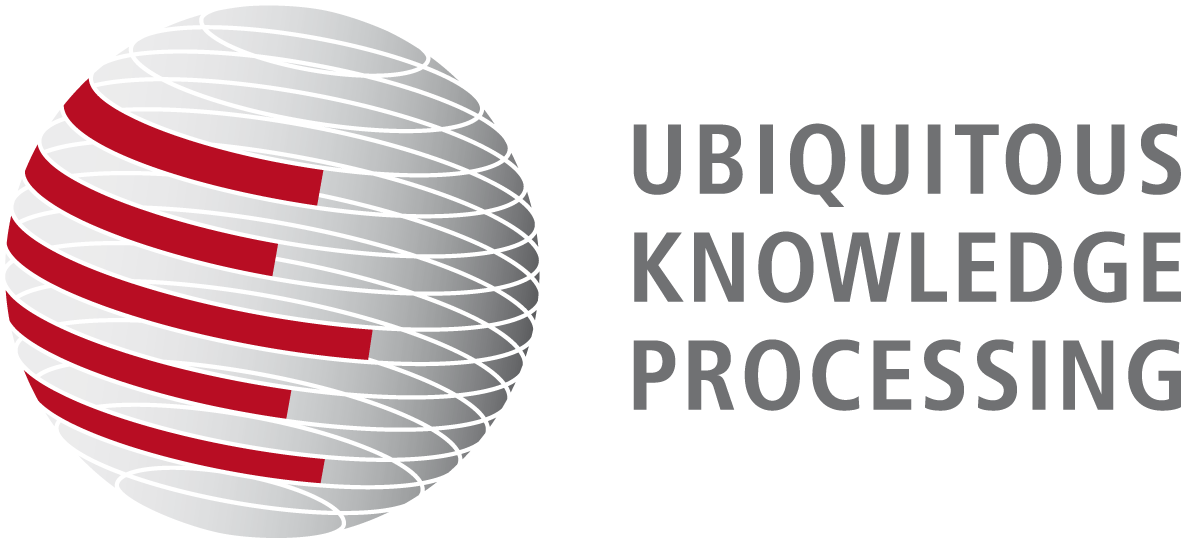