LKE/KDSL Research Seminar
2014/02/28
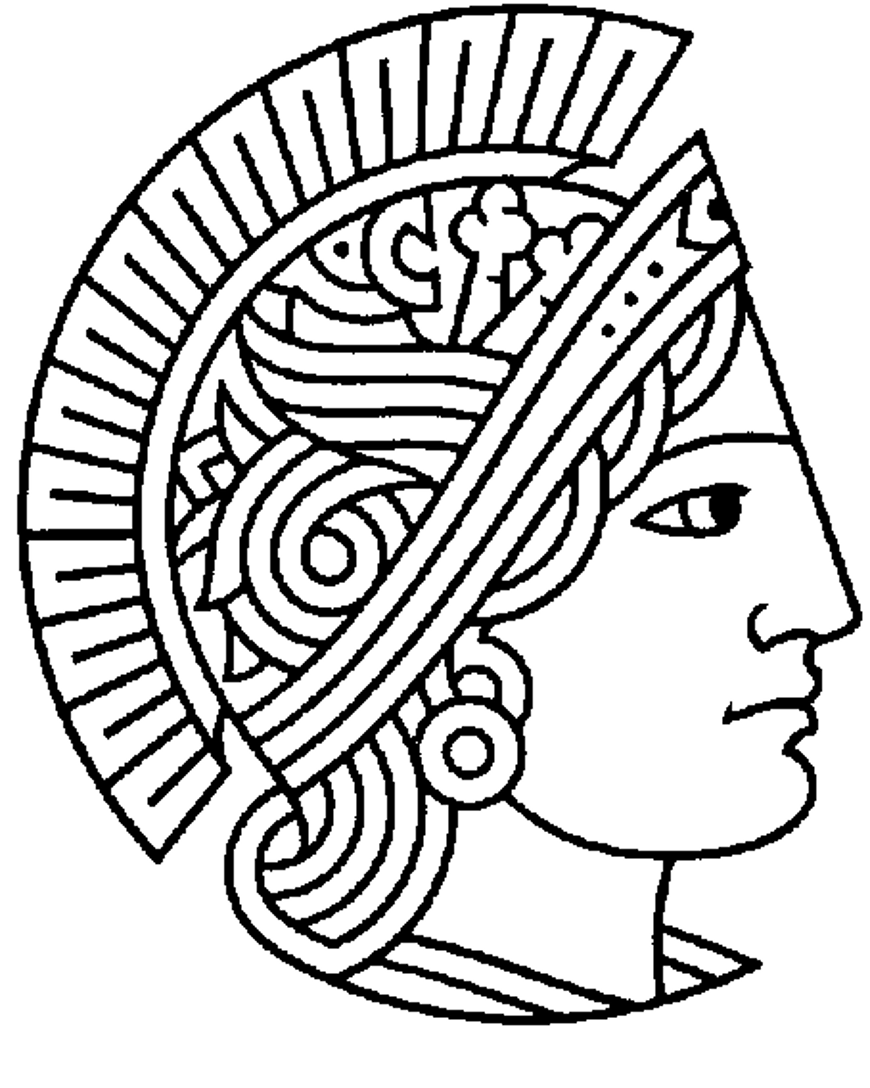
On March 11th, 2014, the LKE/KDSL Research Seminar will host the following talks:
Dr. Ivan Habernal
Title: Argumentation in the Wild: Annotation Study on Argumentation in
User-Generated Content
Abstract: In this talk, I will present our current study on argumentation in user-generated content. Given various controversies in education and a corpus consisting of ~5k documents from discussion forums, comments to articles, and blogs, we investigate micro-level argumentation using Toulmin's argumentation theory. Given various phenomena typical to these registers, we have to deal with pre-selecting candidates suitable for argument annotation (identification of on-topic persuasive texts) as well as to consider multiple dimensions of an argument (i.e. logos and pathos). (Note: this is a work-in-progress report.)
Susanne Neumann:
Title: Identification of Dataset Names in Scientific Literature
Abstract: An increasing amount of scientific literature demands intelligent search methods. As a considerable amount of the literature deals with raw or aggregated empirical data, finding mentions of datasets is of high importance for researchers. Extracting dataset names from text can be defined as a named entity recognition task. This project focuses on extracting specialized named entities from German in the educational sciences domain. The lack of annotated corpora is a major issue, so that methods must work with little or no training data. I will introduce the current state of my approaches for extracting dataset names from German scientific abstracts with a focus on minimally-supervised bootstrapping pattern induction. This method only requires a small number of seeds as examples to iteratively generate patterns for repeatedly extracting new entities.
Tristan Miller:
Title: SiDiM: Lexical substitution for text watermarking
Abstract: We propose a supervised lexical substitution system that does not use separate classifiers per word and is therefore applicable to any word in the vocabulary. Instead of learning word-specific substitution patterns, a global model for lexical substitution is trained on delexicalized (i.e., non lexical) features, which allows to exploit the power of supervised methods while being able to generalize beyond target words in the training set. This way, our approach remains technically straightforward, provides better performance and similar coverage in comparison to unsupervised approaches. Using features from lexical resources, as well as a variety of features computed from large corpora (n-gram counts, distributional similarity) and a ranking method based on the posterior probabilities obtained from a Maximum Entropy classifier, we improve over the state of the art in the LexSub Best-Precision metric and the Generalized Average Precision measure. Robustness of our approach is demonstrated by evaluating it successfully on two different datasets.
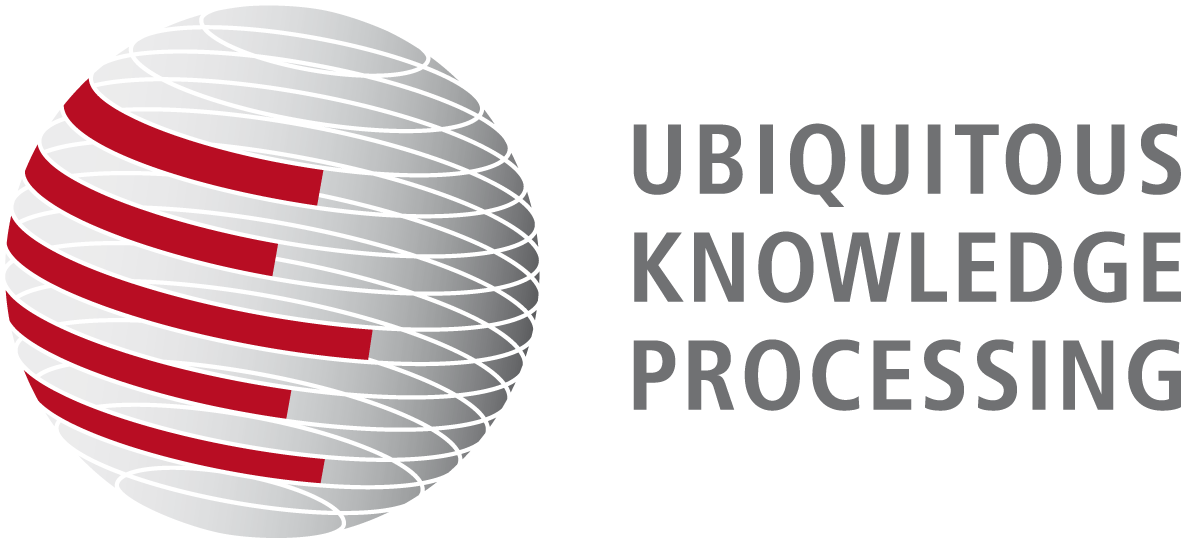