LKE/KDSL Research Seminar
2013/08/19
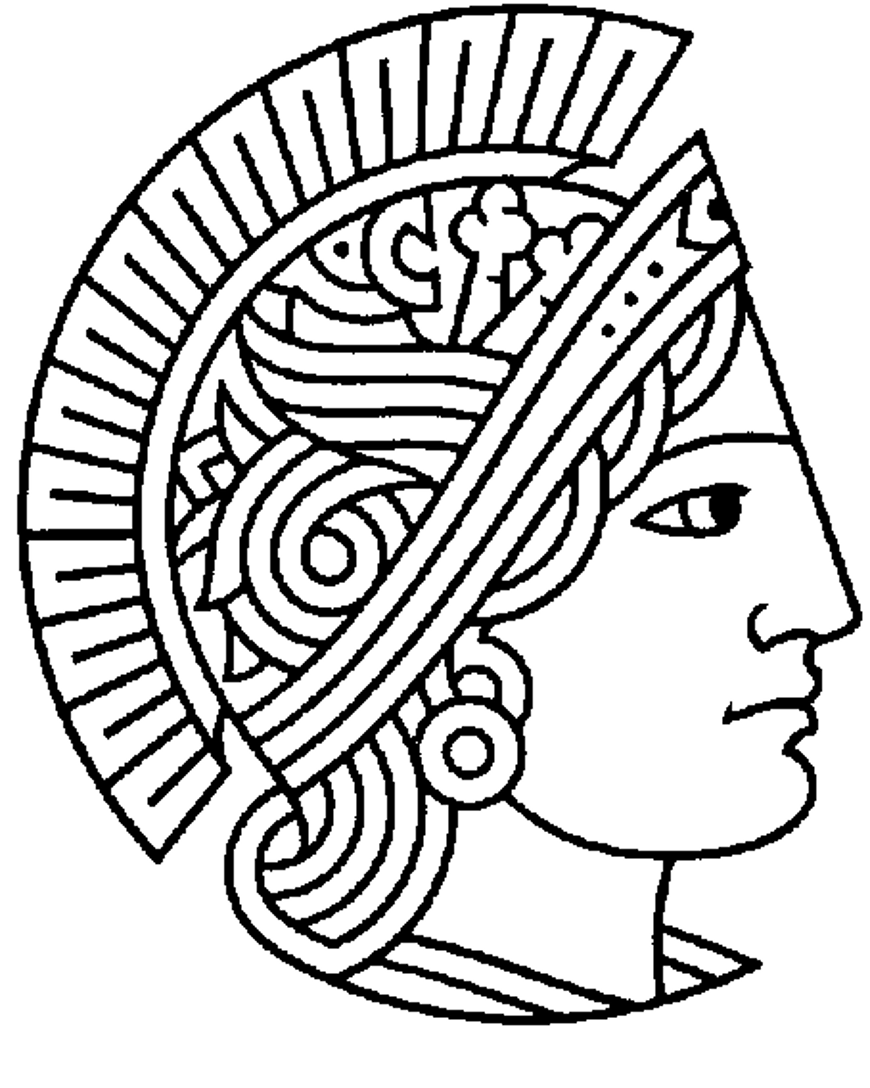
Saurabh Shekhar Verma and Viswanathan Arunachalam will each present talks at the upcoming LKE/KDSL Research Seminar on Tuesday Aug 27, 2013 at 11:40 in S1|03 223.
(S. Verma)
Title: A fine-grained analysis of collaboration in Wikipedia based on edit categories and author networks
Abtract: In this work, we are identifying and analyzing patterns of collaboration among Wikipedia authors. To this aim, we group authors based on the distribution of categories of edits they performed in Wikipedia articles. Having identified groups of authors by clustering, each cluster is labeled with certain role depending on the properties it shows. And, we generated a network of co-authors in which every node is an author (author assigned with a label) and edge is the interaction between them while editing the article. Then we identified frequent collaboration motifs from this network, for qualitative analysis of the articles.
(V. Arunachalam)
Title: Automatic detection of correlated edit-turn pairs from English Wikipedia using Machine Learning
Abtract: The main objective of this thesis is to identify edits from a Wikipedia article’s revision history which are related to problems discussed on the respective Wikipedia Talk page. At present, detecting correlated edits and turns in Wikipedia is considered to be a high impact Human Intelligence Task (HIT) because there is no system which is able to do this. To solve this problem, I build a system which performs preprocessing and feature extraction on an input dataset consisting of edit-turn-pairs from English Wikipedia articles and apply supervised machine learning to detect correlated pairs. I used a Gold Standard corpus based on human annotations to evaluate my features. The Gold Standard corpus comprises of 657 instances containing the edit and turn text and various kinds of metadata information about edit-turn-pairs. The annotators had labeled 128 edit-turn-pairs as positive (i.e., correlated) and the remaining 529 edit-turn-pairs as negative. On applying supervised machine learning with the extracted features from edit-turn-pairs, the best classifier provided an accuracy rate of 0.87. Cosine Similarity, Semantic Similarity and Time Distance were the features that turned out to be very important for the task of finding correlated edits and turns. Potential applications of this system include bootstrapping new samples of correlated edit-turn-pairs and correlating the output of my system to article quality to learn about the connection between collaboration on Talk pages and article quality.
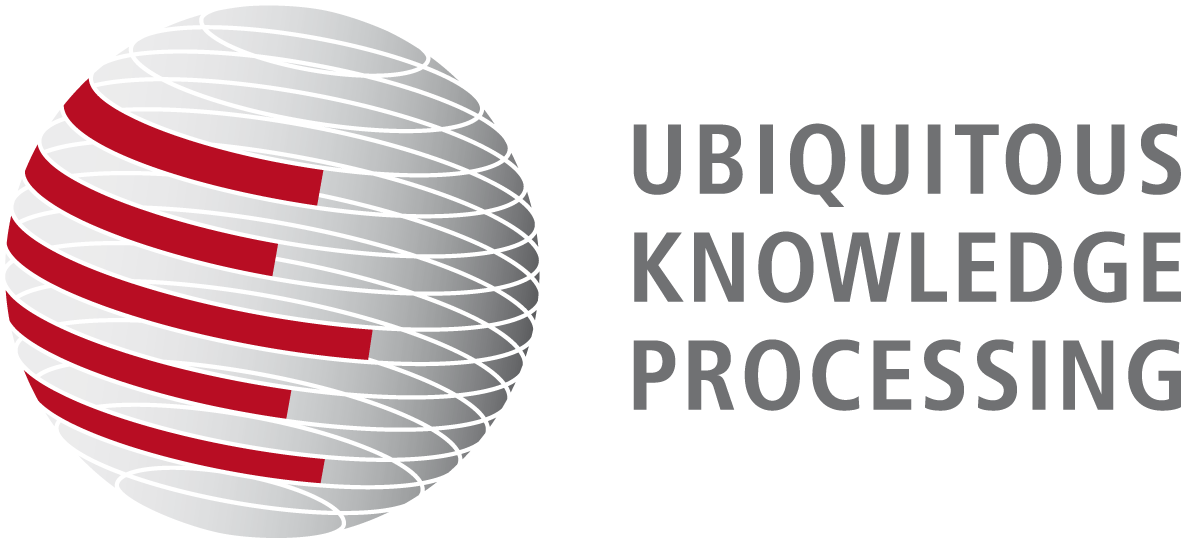