The Least Confident Delivery Drone Gets the Job
Researchers from TU Darmstadt and ccollaborators present study
2025/05/20 by bjp
In the future, autonomous delivery drones could independently assess whether their remaining battery charge is sufficient for upcoming deliveries. A team of researchers from Technical University of Darmstadt and the University of Sheffield, in collaboration with the French National Institute for Research in Digital Science and Technology (INRIA) and industry partner Ingeniarius Ltd, has developed a new method for energy-aware deployment planning. The approach enables each drone to learn what orders it is capable of fulfilling even when not knowing its own battery health. It is shown to reduce delivery times and increase the number of processed orders compared to conventional approaches.
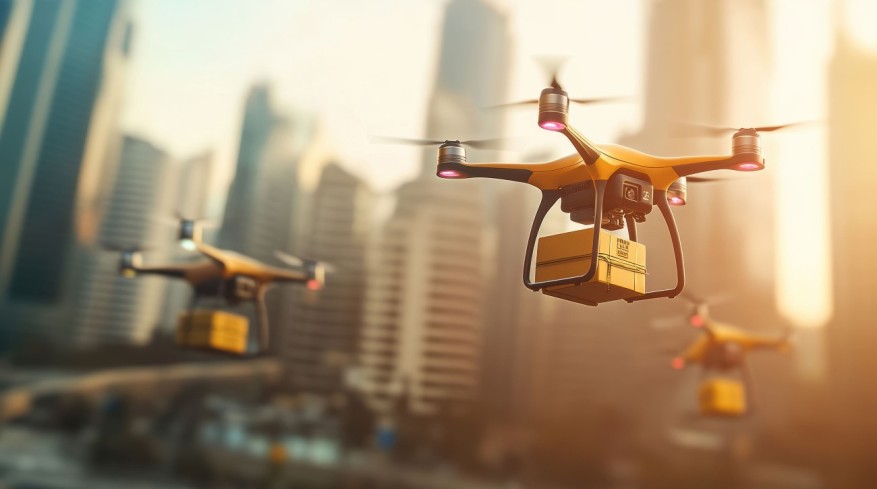
At a fulfilment centre, delivery drones assign tasks among themselves using an auction-based system. Each drone considers its current battery level and evaluates whether it can complete the task. If so, it places a bid that reflects its confidence. The drone that wins the auction attempts the task and uses the outcome to refine its understanding of its true capabilities, which are influenced by unknown factors such as the long-term health of its battery. Counterintuitively, selecting the least confident bidder as the auction winner proved most effective. This approach enabled drones to learn more accurately where their performance limits lie and promotes smarter use of resources by deploying drones whose capabilities are well-matched to the task at hand.
Video about the study
The study was selected as a finalist for the Best Paper Award
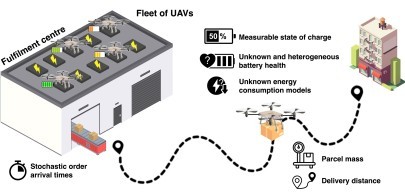
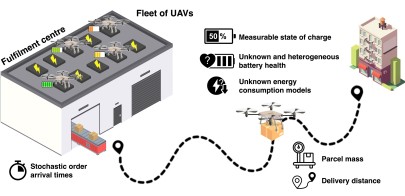
The researchers, led by from the Department of Computer Science at TU Darmstadt, tested their method in a specially developed multi-agent simulator over a period of eight weeks. The results showed that the learning-based approach achieved significantly higher delivery rates and shorter delivery times compared to conventional threshold-based strategies. In an extended version, drones were even able to take on tasks that they could complete only once sufficiently recharged, enabling a forward-looking allocation of resources. ‘This work shows how online learning can help robots cope with real-world challenges, such as operating without full knowledge of their true capabilities,’ said Dr Mohamed Talamali from The University of Sheffield. Professor Roderich Groß
The approach can also be used to efficiently manage heterogeneous fleets in which the drones differ, for example, due to manufacturing tolerances or individual wear and tear. This paves the way for autonomously operating delivery systems with higher reliability and optimised energy usage. ‘Such autonomous delivery drones could also operate across multiple fulfilment centres, further reducing delivery times and costs,’ said Professor Groß.
The study ‘Ready, Bid, Go! On-Demand Delivery Using Fleets of Drones with Unknown, Heterogeneous Energy Storage Constraints’ will be presented on 21 May at the in Detroit, USA, and was selected as a finalist for the Best Paper Award from more than 1,000 submissions. It received funding from the 24th International Conference on Autonomous Agents and Multiagent Systems. Horizon Europe project OpenSwarm