Vision teacher for computer
hoch³ Forschen 4/2017 – science quarterly
2018/05/23 by Christian Meier
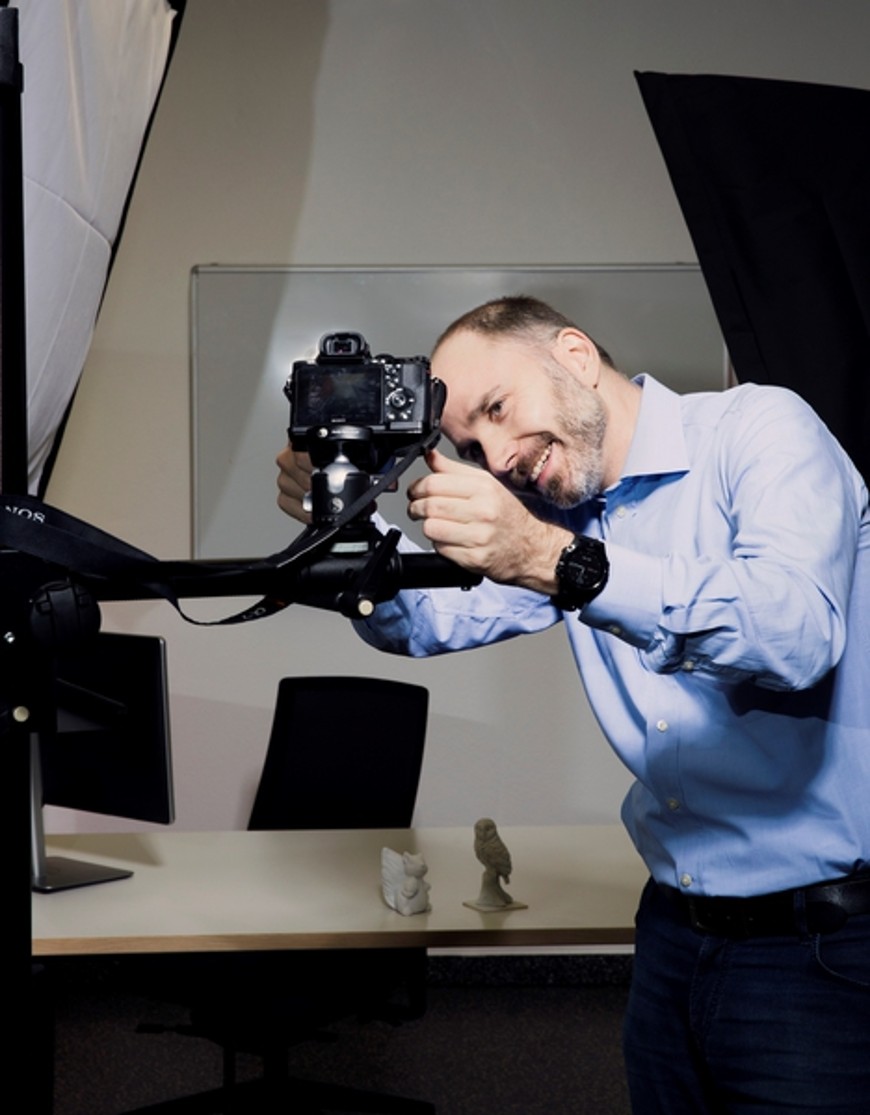
A typical street scene can be seen on the screen in Stefan Roth's office – but from the 'viewpoint' of a computer. Cars tinted red pull in and out of parking spaces, purple pedestrians bustle about, green-marked plants indicate the verge. “For the computer, a video first of all only consists of pixels”, explains . “We teach it to interpret the pixels”, adds the head of the computer science professor Stefan Roth at Technische Universität Darmstadt. Roth's team teaches intelligent algorithms to detect cars, pedestrians, or even potentially dangerous objects in X-ray images from transportation security. The software developed by the scientists of TU Darmstadt also reconstructs the image formation that may be hidden behind blurred or out-of-focus images. The research question that guides them: How much information can be extracted from a digital image? Visual Inference Lab
The need for automatic image analysis is huge. Millions of digitial cameras create an unprecedented flood of images. If computers could reliably interpret not only ordered road scenes such as on a motorway, but also traffic that may appear rather chaotic, for instance at a junction, “then fully automomous driving would also be possible in busy inner cities”, says Roth. “There are many other potential fields of application”, adds the computer scientist. Intelligent image analysis systems could assist users in tedious tasks, such as bagge control at airports. Land use can be automatically classified in satellite images, for example to ascertain on which fields wheat grows.
But teaching computers to see is difficult. Decades ago, researchers tried to directly create programs that imitate human perception. But this was largely unsuccessful, at least so far. “Today's approaches are very data-driven”, says Roth. Computers learn by means of large quantity of examples. The basis are often so-called artificial neural networks. These are inspired by the structure of the brain: Nerve cells, referred to in technical language as neurons, are interconnected by neural pathways. When photos of cars are shown to such a network, recurring patterns such as chassis, wheels, and headlights, reinforce neural pathways. If similar patterns appear on unknown photos, the same neurons become active via the intensified neural pathways as during training: The neural network has learned to recognise cars in images. Or pedestrians and plant pots.
The catch: During training one has to literally show the computer on each sample image where the car is, where the pedestrian is, and where the plant pot is. “This used to take us an hour and a half per image at the beginning”, says Roth. Because computers only reliably recognise objects after ten of thousands of examples, that is not alwys practical. “For this reason, we first of all try to get by with less data and secondly, aim to access data sources that already contain some of the information”, says Roth. Computer games, for instance, show deceptively realistic street scenes. On a photo of a real scene, the researchers first have to painstakingly separate the individual objects from each other by tracing their outlines. “In a computer game, however, the individual objects are already separated”, explains Roth. Then one only has to tell the neural network where the cars and the road surface are. [more]
You can read the complete article in the – the science quarterly. current issue of hoch³ FORSCHEN