Combating hunger with artificial intelligence
Computer scientists want to improve the world food conditions
2018/06/21 by Boris Hänßler
Almost 800 million people worldwide suffer from malnutrition. In the future there could be around 9.7 billion people – around 2.2 billion more than today. Global demand for food will increase as climate change leaves more and more soil infertile. How should future generations feed themselves?
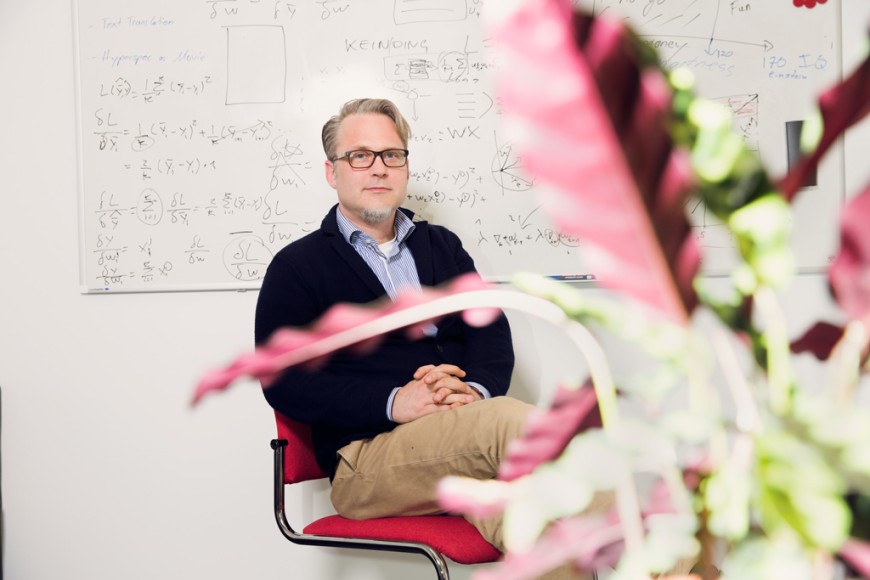
Kristian Kersting, Professor of Machine Learning at the TU Darmstadt, and his team see a potential solution in the application of artificial intelligence (AI). Machine learning, a special method of AI, could be the basis for so-called precision farming, which could be used to achieve higher yields on areas of equal or smaller size. The project is funded by the Federal Ministry of Food and Agriculture. Partners are the Institute of Crop Science and Resource Conservation (INRES) at the University of Bonn and the Aachen-based company Lemnatec.
“First of all, we want to understand what physiological processes in plants look like when they suffer from stress”, said Kersting. “Stress occurs, for example, when plants do not absorb enough water or are infected with pathogens. Machine learning can help us to analyse these processes more precisely.” This knowledge could be used to cultivate more resistant plants and to combat diseases more efficiently.
Clever solution for data analysis
The researchers are currently working on teaching the software to optimise itself using deep learning and to find the patterns that represent stress more quickly. “A healthy spot can for instance be identified from the chlorophyll content in the growth process of the plant”, said Kersting. “When a drying process occurs, the measured spectrum changes significantly.” The advantage of machine learning is that it can recognise such signs earlier than a human expert, as the software learns to pay attention to more subtleties.
The researchers are currently working on teaching the software to optimise itself using deep learning and to find the patterns that represent stress more quickly. “A healthy spot can for instance be identified from the chlorophyll content in the growth process of the plant”, said Kersting. “When a drying process occurs, the measured spectrum changes significantly.” The advantage of machine learning is that it can recognise such signs earlier than a human expert, as the software learns to pay attention to more subtleties.
It is hoped that someday, cameras can be installed along rows of plants on an assembly line in the greenhouse, allowing the software to point out abnormalities at any time. Through a constant exchange with plant experts, the system should also learn to identify even unknown pathogens. “Ultimately, our goal is a meaningful partnership between human and artificial intelligence, in order to address the growing problem of world nutrition”, says Kersting.