Continual Cross-Lingual Multimodal Retrieval
Master Thesis
Fine-tuning a pre-trained language model (PLM) on downstream tasks has been a standard approach in NLP. However, PLMs suffer from catastrophic forgetting when adapting to a sequence of tasks. In a real-world scenario, data is collected in a stream fashion – particularly in the multimodal (image and text) multilingual setting, where new visual concepts can emerge and new languages can be incorporated later on, which can result in catastrophic forgetting. In this thesis, we aim to 1) understand if cross-lingual multimodal retrieval suffers from catastrophic forgetting, 2) how the continual learning setting affects changes in representations, 3) if current continual learning methods alleviate catastrophic forgetting in cross-lingual multimodal retrieval, 4) how to design new methods to alleviate catastrophic forgetting in the problem setup.
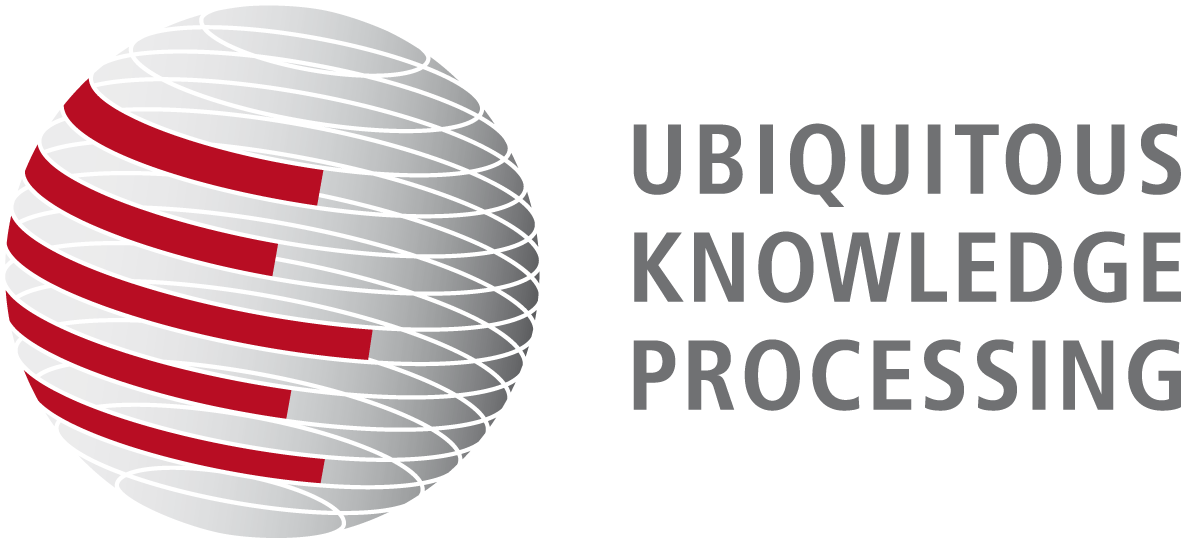